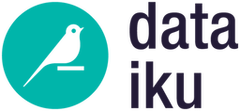
AI in automotive: capturing value beyond (just) the vehicle
Continue reading
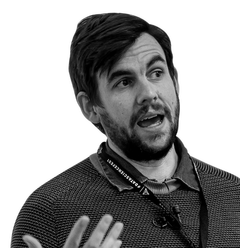
Shaun McGirr, Field Chief Officer, Dataiku
What is AI and is Generative AI any different?
To this day, academics argue the definition of artificial intelligence (AI). Still, in their book “Prediction Machines”, three University of Toronto economists explained that AI refers to machines capable of learning from data, in order to make predictions or take actions based on that learning. The authors offer another definition that will be relevant to anyone in business: AI is a cheap way of making accurate predictions. And whenever something becomes cheaper, we engage it more often.
In short, AI is just the latest technology helping us predict better and faster, which hints at how its latest wave, "Generative AI”, might play out. Based on large datasets, the most well-known example, ChatGPT, has radically reduced the cost of predicting the next best word in a sentence to the point that it can effectively mimic human communication. It is best applied to problems where summarising large bodies of text is a relevant solution. The good news is that plenty of such use cases exist throughout automotive.
"Manufacturers have decades of experience putting technology in the vehicle but are relatively behind other industries in making their data investments pay off across the entire company."
Shaun McGirr, Field Chief Officer, Dataiku
Was AI oversold in automotive?
When I worked in automotive (2016-2021), it felt like self-driving cars were always just around the corner. Recall the bullish predictions of Elon Musk and others at the launch of Tesla's first models. Contrast that with the sustained difficulties guaranteeing the safety of fully autonomous vehicles that have since emerged. It seems the closer we get, the further the target moves.
Early on, the challenge seemed to be data. But every manufacturer of autonomous systems is no longer short of data. The simple fact is that roads are complex systems driven by interactions between hundreds of individuals (drivers, cyclists, pedestrians) pursuing various objectives (speed, safety) in constantly changing environments (weather, traffic, road condition). Increasing autonomous driving is more about controlling these inputs than refining self-driving algorithm outputs.
Thankfully, as often happens with innovation, the 'moonshot' application (self-driving cars) has spawned several more mundane applications that are now standard: lane-keep assist, radar-assisted cruise control and all manner of warning systems. These add value precisely because they help the driver in the most challenging circumstances (heavy traffic, bad weather) rather than replacing the driver when their job is the easiest (on straight and empty highways).
Another spin-off benefit has been the richer data collected about everything from traffic to driver behaviour through to vehicle performance, which presents challenges. Manufacturers have decades of experience putting technology in the vehicle but are relatively behind other industries in making their data investments pay off across the entire company.
Value of AI beyond the vehicle
If we set aside AI for autonomous driving, how can the incredible wealth of data generated by connected vehicles be valuable? Beyond the obvious operational use cases (fleet location, predictive maintenance), a significant value is currently ripe for use.
Let's start with the product development process itself. An incredible amount of institutional knowledge is tied up in the minds of current designers and engineers. Still, many of their workflows have not changed fundamentally in decades, even if they were digitised. The good news is that all that know-how, the essence of a brand's value, is contained in the history of all the product development that’s been completed.
Dataiku's customer GE Aviation used this insight years ago to cut product design times in half while increasing fuel efficiency by 7% in a piston engine. Their AI system used the results of previous (and costly) full-blown simulations of the part in question to train a neural network that could predict the simulation results of potential designs. Such a drop in the cost of predicting successful designs allowed them to do much more: pre-testing millions of potential designs in minutes, distilling all that human knowledge and discovering an edge. This approach is already widespread in pharmaceutical and materials design but has incredible potential in automotive to reduce the weight of every part while increasing its strength.
Moving through the manufacturing process, let's skip over the factory floor… where it's already been digitised for decades and automated beyond recognition, with a whole host of AI-powered robots. In this area, automotive has truly led the way and those innovations have now seeped into every other heavily manual process, from packing groceries to bomb disposal.
I see the most untapped potential for the whole automotive industry in (re)using data from everyday operations, and connected vehicles, to improve how everything runs outside the factory. I recall a disturbing conversation with the UK used car manager of a large German manufacturer in 2017. He simply wanted to know which factory options were installed on each vehicle for disposal by his company. Cox Automotive, a third party, had more accurate data for that question than he did. I want to say the situation must have changed, but it represents an approach to data that belies the persistence of 20th-century siloes across the industry. Those were built for good reasons: clear contracting and accountability between the many links in very complex supply chains.
Today's world is different, from geopolitical tension over semiconductors to the lingering effects of COVID, the only way for any automotive player to get an edge is to predict a challenge before it hits them, creating time to react. Restricting data to the silo that created it scales the problem I encountered in 2017. It turns out that finance needs to estimate sales, production and supply chain pressures just to manage cash flow and as the world returns to travel, previous approaches to predicting rental car demand need reinvention and daily recalibration.
Recommendations
The blinding light of ChatGPT has put AI on everyone's agenda and illuminated many shadows where even decades-old AI technology could already be making a difference. Perhaps it wasn’t applied due to inertia and the comfort of old business models.
As the industry confronts multiple regulatory and economic challenges while advancing autonomous driving and electrification, it must not be too proud to admit it is a little behind. It should use the rapidly dropping cost of predictions to change decisions. In its favour is the extraordinary wealth of experience and knowledge, which, if made more flexible in combination with AI, will not replace humans but make them even more powerful.